The Age of Agentification: Navigating AI Integration for Long-Term Success
- Sam Leigh
- Mar 17
- 7 min read
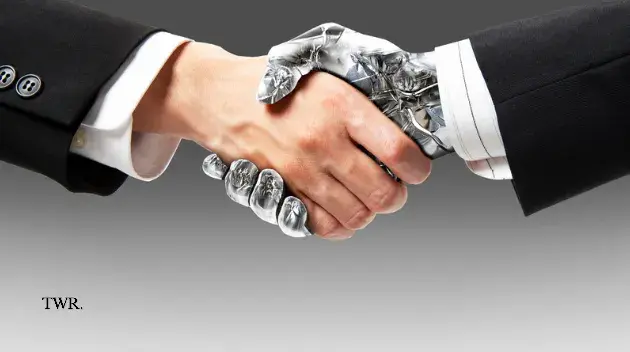
by Sam Leigh | March 17, 2025
In boardrooms across the globe, one conversation is dominating agendas: the transformative potential of artificial intelligence. From OpenAI’s generative GPT agents to NVIDIA’s Nanotron models and Google’s Gemini 2.0, the world is on the cusp of a revolution in how organizations operate. But behind the buzzwords like “agentification” and “AI orchestration” lies a sobering truth — AI is not a plug-and-play solution. Effective adoption requires more than access to cutting-edge platforms. It demands a carefully calibrated strategy, and for many organizations, that means finding the right partner.
The challenge for businesses is not in deciding whether to adopt AI but in determining how to do so. Integrating AI agents into workflows, processes, and decision-making is a monumental task. It’s one that involves technical complexity, ethical considerations, and long-term planning. For many organizations, the only viable path forward is to engage with intermediaries who act as stewards of transformation, helping them navigate the rapidly evolving AI ecosystem.
Key Insights
Strategic AI Integration Requires Expertise: Effectively adopting AI demands a comprehensive approach that balances technical innovation with operational redesign — far beyond simply implementing tools.
Avoid the Pitfalls of Vendor Dependency: Independent partners ensure organizations retain flexibility and autonomy, helping navigate the complexities of multi-platform ecosystems while safeguarding data control.
Navigating Ethical and Workforce Shifts: From ensuring compliance and mitigating bias to upskilling teams and fostering cultural alignment, expert guidance is essential to handle the ripple effects of AI adoption.
Partnering for Success: Collaborating with a dedicated intermediary ensures that the organization’s unique goals remain central, bridging the gap between platforms and enterprise needs while driving sustainable transformation.
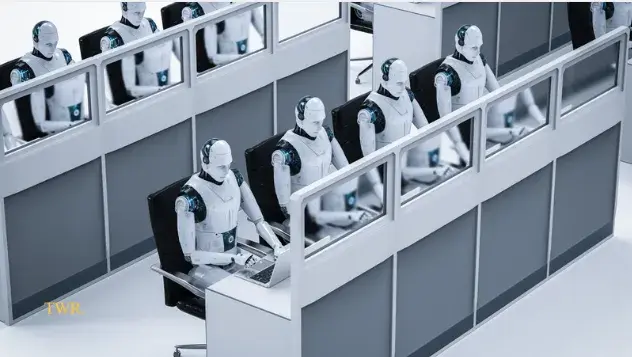
“Agentify Me": A New Era of Productivity
Agentification is the practice of deploying AI systems — often called “agents” — to perform tasks autonomously. Unlike traditional software, these agents can learn, adapt, and make decisions, often exceeding human capabilities in speed and scale. From automating customer service interactions to predicting supply chain disruptions, the applications are endless.
For example, Google’s Gemini agents are transforming conversational interfaces, making them more responsive and intuitive. NVIDIA’s Nanotron models aim to revolutionize workflow automation in industries like healthcare and logistics. These tools promise unprecedented efficiencies, but they also introduce significant complexity. Organizations are quickly realizing that adopting these systems involves far more than flipping a switch.
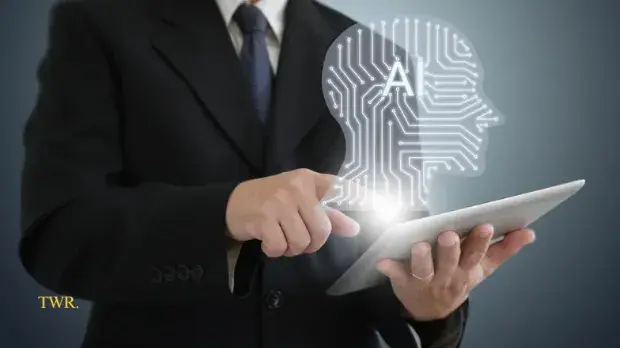
The Need for Strategic Partnerships
AI platforms are often designed to solve specific problems, but they rarely integrate seamlessly into an organization’s existing systems. This is where many companies falter. They underestimate the effort required to unify disparate tools, retrain staff, and align AI capabilities with broader business objectives.
More importantly, relying on platform providers to guide this transition can be risky.
These companies have an inherent interest in promoting their tools, not in addressing the nuanced needs of the organizations adopting them.
To bridge this gap, businesses increasingly turn to external partners — neutral advocates who specialize in managing relationships with AI vendors, optimizing implementations, and ensuring that the organization’s interests come first.
A Case Study in Transformation: Streamlining Global Logistics
Consider a global logistics company, “Atlas Transport,” facing mounting pressures from rising operational costs and market competition. Eager to modernize, the company signed contracts with two leading AI platforms — one focused on predictive analytics for inventory management and another specializing in route optimization.
Initially, these tools delivered results in their silos: the inventory platform improved forecasting accuracy by 15%, while the route optimization tool reduced delivery times by 10%. But the lack of integration between these systems created bottlenecks. Drivers received route updates that didn’t align with warehouse inventory data, leading to delays and lost revenue. Leadership began to doubt whether their investment in AI was worth the hype.
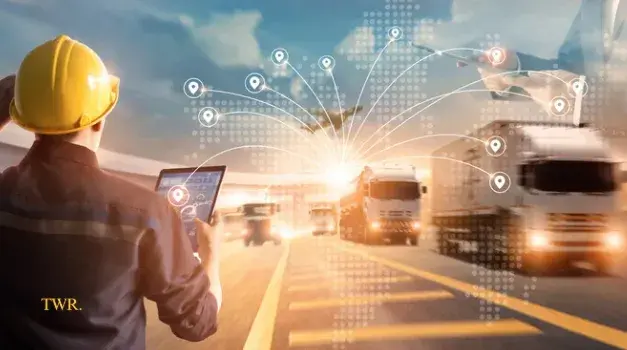
Recognizing the need for a more cohesive approach, Atlas engaged an external partner — a firm specializing in AI orchestration and agent management. The partner didn’t just integrate the two systems; they took a holistic view of Atlas’s operations, reworking workflows to align with AI-generated insights. They implemented middleware to unify data flows between platforms and retrained employees to adapt to AI-enhanced processes.
The result? A 30% reduction in operational costs and a 20% increase in on-time deliveries within six months. Just as importantly, the leadership team regained confidence in their AI strategy, knowing they had a partner focused solely on their success, free from the biases of platform providers.
The Hidden Complexity of AI Integration
The Atlas Transport case serves as a vivid example of how successful agentification isn’t merely about acquiring AI tools — it’s about fundamentally rethinking the operational architecture of organizations. AI systems introduce disruptions that challenge existing structures, redistribute labor responsibilities, and redefine success metrics. Below, we delve deeper into the hidden complexities of AI integration and the pillars that determine its success.
1. Navigating Platform Ecosystems
The AI ecosystem is fragmented and proprietary by design. Major players like OpenAI, NVIDIA, and Google each bring distinct capabilities to the table, but they also build tools tailored to specific applications, with unique technical requirements and limited interoperability.
Strengths and Limitations: OpenAI’s generative GPT agents excel in natural language processing, while NVIDIA’s Nanotron models lead in workflow automation and real-time analytics. Google’s Gemini agents shine in conversational AI and data processing at scale. However, integrating these tools to work harmoniously requires expertise. Mismatched systems or a lack of interoperability can result in data silos, bottlenecks, and inefficiencies.
Integration Challenges: The seamless use of multiple AI platforms often hinges on middleware — custom software designed to connect disparate tools. Without proper integration, AI systems may operate in isolation, undermining their potential impact.
Strategic Tool Selection: Businesses must evaluate platform ecosystems based on their specific needs, not the sales pitches of vendors. This requires deep technical assessments and a clear understanding of how each platform aligns with broader business goals.
2. Avoiding Vendor Lock-In
While platform providers are critical enablers of AI adoption, they are also businesses with their own interests. Their goal is to secure long-term clients within their proprietary ecosystems, which can lead to vendor lock-in — a situation where organizations become overly dependent on a single provider, limiting flexibility and increasing costs.
Risks of Lock-In: Vendor lock-in can stifle innovation by making it difficult to adopt new technologies outside the chosen ecosystem. It also risks data portability issues, where moving data to another platform becomes cumbersome or costly.
Maintaining Flexibility: Independent partners help organizations remain agile by designing adaptable AI architectures. This includes selecting open-source tools when possible, advocating for contracts that ensure data portability, and establishing contingencies for transitioning to new providers.
Cost Implications: Vendor lock-in can escalate costs over time as organizations are forced to pay for updates, additional features, or integrations that might otherwise be avoidable in a more flexible system.
3. Ethical and Regulatory Challenges
As AI adoption accelerates, organizations face increasing scrutiny over how they manage ethical and regulatory risks. Issues like data privacy, algorithmic bias, and compliance with global regulations are not just legal concerns — they are reputational risks that can undermine trust.
Data Privacy: AI systems rely on vast amounts of data, raising concerns about how sensitive information is collected, stored, and used. In regions with stringent privacy laws like GDPR (Europe) or CCPA (California), compliance is non-negotiable.
Algorithmic Bias: Bias in AI models can lead to discriminatory outcomes, whether in hiring processes, credit scoring, or customer service. Partners with expertise in ethical AI can help organizations audit algorithms, retrain models, and ensure fairness.
Global Compliance: Regulations vary widely across jurisdictions. For example, Europe prioritizes data sovereignty, while China focuses on national security in AI applications. Partners play a critical role in navigating these complexities, ensuring organizations remain compliant while leveraging AI’s full potential.
4. Workforce Transformation
AI adoption doesn’t just automate tasks — it redefines roles, workflows, and organizational culture. While businesses focus heavily on the technical side of AI integration, they often underestimate the human impact.
Upskilling Employees: Workers need new skills to interact effectively with AI systems, from interpreting AI-generated insights to managing hybrid workflows where humans and agents collaborate. This requires robust training programs and a commitment to continuous learning.
Redesigning Processes: AI introduces efficiencies, but it also disrupts established workflows. Processes that once relied on manual decision-making must be re-engineered to align with AI’s capabilities, ensuring smooth handoffs between human and machine.
Cultural Adaptation: Resistance to change is a natural human reaction. Leaders must foster a culture of innovation, emphasizing how AI augments rather than replaces human roles. Transparency, communication, and inclusivity are critical to gaining employee buy-in.
Metrics for Success: Traditional performance metrics may no longer apply in AI-enhanced environments. Organizations need to define new benchmarks for success that reflect both human and machine contributions, ensuring accountability and alignment with strategic objectives.
Beyond the Basics: Why Strategic Partnerships Matter
The complexities outlined above demonstrate that AI integration is not a do-it-yourself endeavor. Without expert guidance, organizations risk falling into common traps: overspending, underutilizing tools, and failing to achieve the transformative impact they seek.
Strategic partners act as navigators in this landscape. They bring impartial expertise, advocating for the organization’s interests over those of platform providers. They offer comprehensive solutions that go beyond tool selection, addressing the operational, cultural, and ethical dimensions of AI adoption.
For organizations ready to embrace agentification, the right partner can mean the difference between incremental improvements and a fundamental transformation. As the age of AI unfolds, success will belong to those who approach this new frontier with strategy, foresight, and the right alliances.
Looking Ahead: The Future of Agentification
Agentification is reshaping industries at a breathtaking pace, from healthcare to finance, retail to logistics. But its success hinges on more than the technology itself. It requires vision, strategy, and execution.
For organizations navigating this new landscape, the message is clear: don’t go it alone. The right partner can turn the promise of AI into a reality, transforming not just workflows but the very fabric of how businesses operate.
As the Age of Agentification unfolds, the question is not whether organizations will adopt AI agents, but how effectively they will do so. And for those ready to rise to the challenge, the rewards will be extraordinary.
Comments